Response Modeling Methodology: Empirical Modeling for Engineering and Science
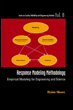
RMM provides platforms for relational modeling and for modeling random variation. The former provides models which incorporate both transmitted variation (systematic and random) and self-generated variation (random). In Chapter 8 estimation procedures for this type of models were developed.
To model random variation, the component of systematic variation in the RMM model is assumed to vanish [the linear predictor (LP) is constant], and the resulting RMM error distribution is then employed as a general platform to model diversely-shaped distributions. Estimation procedures for the parameters of the RMM error distribution are developed in this chapter.
Setting the LP arbitrarily to one, the four variations of the quantile function of the RMM error distribution, corresponding to the four variations in Section 7.2.5 (see also Section 9.4), are
(11.1a) | ![]() |
(11.1b) | ![]() |
(11.1c) | ![]() |
(11.1d) | ![]() |
where (11.1a) corresponds to the original RMM model, derived axiomatically in Chapter 7.
Assume that the errors, ? 1 = ? ?1Z 1 and ? 2 = ? ?2Z 2, originate in a bi-variate normal distribution with correlation ?, or, equivalently, that Z 1 and Z 2 are from a bi-variate standard normal distribution with correlation ?. By conditioning Z 2 Z 1=z 1, the errors can be re-written as
(11.2a) | ![]() |
where Z 1 and Z 2 are now independent standard normal variables.
Similarly, by conditioning Z 1 Z 2=z 2, we have
(11.2b) | ![]() |
where Z 1 and...